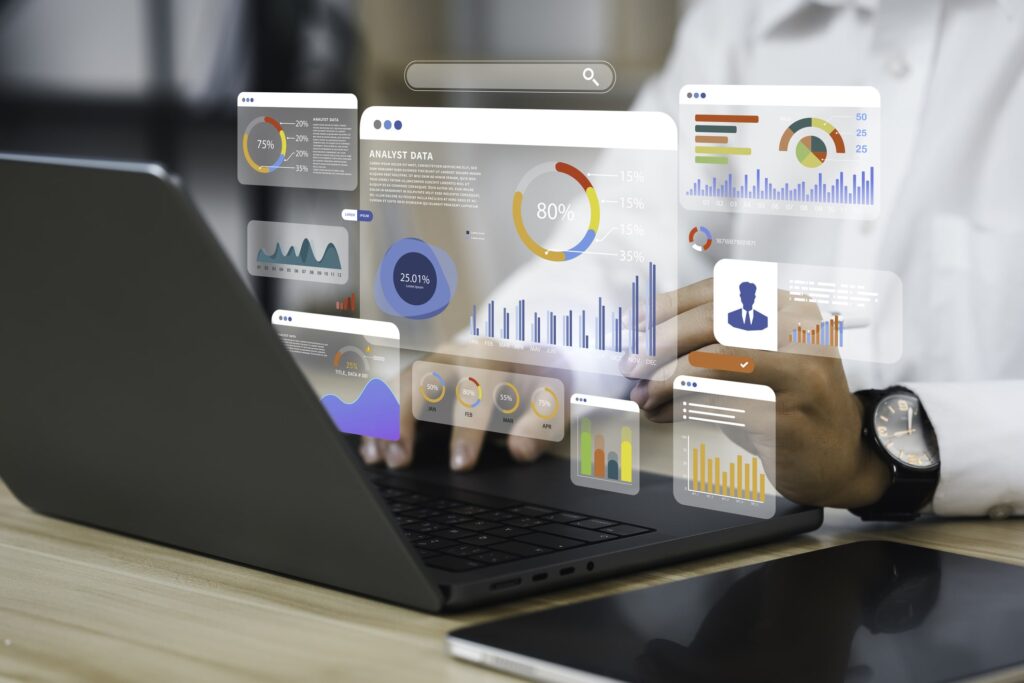
You’re looking at mountains of marketing data, trying to figure out what your customers really want. Making sense of all these numbers and turning them into real marketing results feels overwhelming. AI marketing analytics can help you cut through this complexity and make decisions that work.
That’s exactly why I’ve created this comprehensive guide. We’ll explore everything from basic AI marketing concepts to advanced techniques that’ll give you a competitive edge. You’ll learn how to implement predictive analytics, automate your decision-making processes, and create personalized customer experiences that work.
This guide will show practical ways to use AI marketing analytics for better results. You’ll learn how to improve your campaigns, understand your customers better, and make your marketing budget work harder. Whether you’re new to AI or already using basic analytics, you’ll find clear steps to move forward.
Table of Contents
Understanding AI Marketing Analytics Fundamentals
What is AI Marketing Analytics?
At its core, AI marketing analytics combines artificial intelligence and machine learning with traditional marketing metrics to uncover deeper insights from your data. Unlike conventional analytics tools that simply track and report numbers, AI Marketing tools actively learn from patterns in your marketing data to predict trends and suggest optimizations.
Think of AI marketing analytics as your personal data scientist, working 24/7 to analyze customer behaviors, campaign performance, and market trends. It goes beyond answering “what happened?” to tell you “why it happened” and “what’s likely to happen next.”
Core Components of AI Marketing Analytics
The foundation of AI marketing analytics rests on three interconnected layers that work together to deliver actionable insights. The data collection layer serves as the foundation, gathering information from multiple channels and processing it in real time. This layer ensures that your data is clean, verified, and ready for analysis.
The analysis layer builds upon this foundation, employing sophisticated pattern recognition algorithms and machine learning models. This is where the magic happens – transforming raw data into meaningful patterns and predictions. Natural language processing capabilities allow for an understanding of customer sentiments and preferences across various communication channels.
The insight generation layer represents the final transformation of data into actionable business intelligence. Here, automated reporting systems create easy-to-understand visualizations and recommendations. The system continuously monitors anomalies and identifies emerging trends, allowing for proactive rather than reactive marketing decisions.
Essential Metrics for Modern Marketing
Understanding and tracking the right metrics is crucial for success in AI-powered marketing. Customer-centric metrics tell the story of your audience’s journey and behavior. Customer Lifetime Value (CLV) helps predict future revenue streams, while Customer Acquisition Cost (CAC) ensures your marketing spend remains efficient. Engagement scores and churn probability predictions allow for proactive customer relationship management.
Campaign performance metrics take on new meaning with AI analytics. Multi-channel attribution moves beyond simple last-click models to understand the complex customer journey. Content performance prediction helps optimize your content strategy before publication, while conversion path analysis reveals the most effective routes to purchase.
Real-World Applications of AI Marketing Analytics
The practical applications of AI marketing analytics span across various business domains. In e-commerce, AI analytics drives personalized product recommendations and optimizes pricing strategies based on real-time market conditions. The technology predicts product demand patterns and helps maintain optimal inventory levels, reducing costs while maximizing sales opportunities.
Content marketing benefits from AI’s ability to analyze vast amounts of data to predict content performance. The system identifies topic clusters that resonate with your audience and determines optimal publishing schedules. By analyzing audience preferences at scale, AI helps create content strategies that consistently engage and convert.
Social media marketing reaches new levels of sophistication with AI analytics. Real-time sentiment analysis helps brands maintain their reputation and adjust messaging on the fly. Trend prediction capabilities ensure your content stays relevant and timely, while engagement optimization helps maximize the impact of every post.
Overcoming Implementation Challenges
The journey to implementing AI marketing analytics comes with its share of challenges, but understanding them helps in preparation. Data quality issues often present the first hurdle. Inconsistent formats, missing information, and integration problems can impact analysis accuracy. Establishing robust data governance practices and regular quality checks helps maintain data integrity.
Technical challenges require careful consideration during implementation. Integration with existing systems needs thorough planning to avoid disruption to ongoing operations. Staff training becomes crucial for successful adoption, while resource allocation must balance immediate needs with long-term scalability
Best Practices for Success
Success with AI marketing analytics requires a strategic approach. Begin by establishing clear objectives that align with your business goals. Define specific success metrics and create realistic implementation timelines. This foundation ensures that your AI analytics initiative delivers measurable value.
Data quality management should become a continuous process rather than a one-time effort. Regular audits and cleaning procedures help maintain the accuracy of your insights. Privacy compliance checks protect your customers’ data while maintaining their trust.
Focus on generating actionable insights rather than collecting data for its own sake. Create clear reporting structures that facilitate quick decision-making. Establish feedback loops to continuously improve your analytics processes and adjust strategies based on performance data.
Advanced AI Analytics Techniques
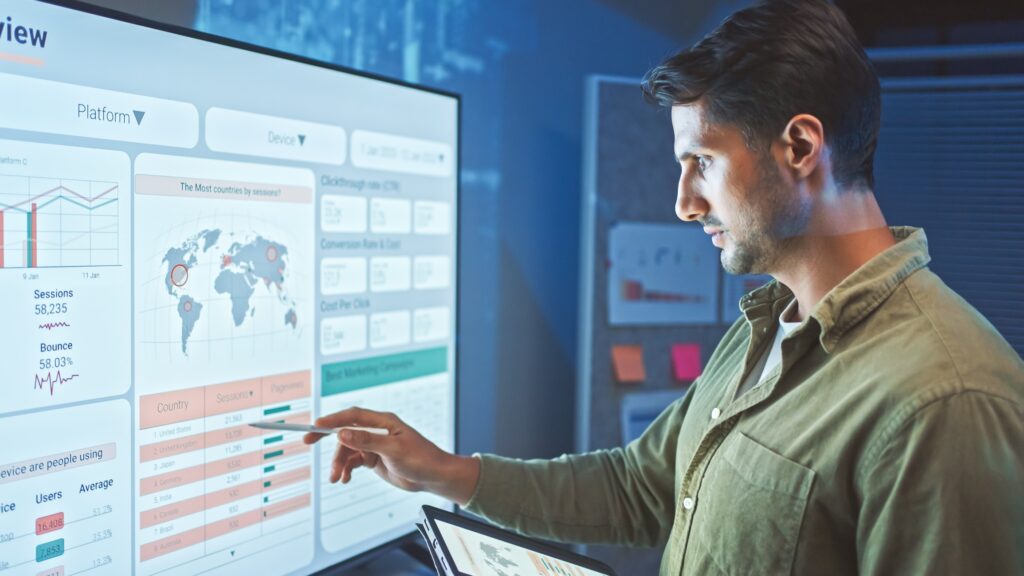
The landscape of advanced AI analytics opens up powerful possibilities for marketing professionals who are ready to move beyond basic metrics. These sophisticated techniques enable deeper insights and more accurate predictions that can transform your marketing strategy.
Predictive Analytics and Forecasting Models
Predictive analytics represents one of the most powerful applications of AI in marketing. These systems analyze historical data patterns to forecast future trends and outcomes. The technology goes beyond simple trend analysis, incorporating multiple variables to create sophisticated forecasting models.
Marketing teams can leverage predictive analytics to anticipate customer behaviors, forecast sales trends, and optimize campaign timing. For instance, the system might analyze seasonal trends, customer behavior patterns, and market conditions to predict optimal launch times for new products or campaigns.
The real power lies in the ability to combine multiple data sources. When you integrate CRM data with social media insights and website analytics, predictive models can identify subtle patterns that humans might miss. These insights help optimize everything from email send times to content recommendations.
Machine Learning for Customer Segmentation
Traditional customer segmentation relies on basic demographics and broad behavioral categories. Machine learning elevates this practice by identifying complex patterns and creating dynamic segments that evolve with customer behavior.
These advanced segmentation models consider hundreds of variables simultaneously, creating micro-segments based on subtle behavior patterns. The system might recognize that customers who browse your website at certain times, read specific content combinations, and interact with particular email campaigns are more likely to make high-value purchases.
Dynamic segmentation adjusts in real time as customer behaviors change. This allows for more personalized marketing approaches and better resource allocation. Your campaigns can automatically adapt to changing customer preferences and market conditions.
Attribution Modeling with Artificial Intelligence
Modern customer journeys rarely follow a linear path. AI-powered attribution modeling helps understand the complex web of touchpoints that lead to conversions. These models go beyond traditional last-click or first-click attribution to provide a more nuanced view of your marketing effectiveness.
AI attribution systems analyze the entire customer journey, weighing the impact of each interaction. They consider factors like the time between touchpoints, the sequence of interactions, and the relationship between different channels. This provides a more accurate picture of which marketing efforts truly drive conversions.
The system continuously learns and adjusts its attribution weights based on new data. This dynamic approach ensures your attribution model stays accurate as customer behaviors and market conditions change. You can use these insights to optimize your marketing mix and budget allocation.
Natural Language Processing for Social Media Analysis
Social media presents a vast source of unstructured data about your customers’ preferences and opinions. Natural Language Processing (NLP) helps make sense of this data by analyzing conversations, comments, and reviews at scale.
Modern NLP systems understand context and nuance in customer communications. They can detect sarcasm, identify emotional undertones, and understand industry-specific terminology. This enables more accurate sentiment analysis and a better understanding of customer feedback.
These systems also help identify emerging trends and potential issues before they become widespread. By analyzing patterns in social media conversations, you can spot potential PR issues, identify new market opportunities, or gauge reactions to your latest campaigns.
A/B Testing with AI Optimization
AI transforms traditional A/B testing into a more sophisticated and efficient process. Instead of testing simple variables one at a time, AI-powered systems can test multiple variables simultaneously and identify complex interactions between different elements.
These systems use machine learning to optimize testing parameters in real time. Rather than waiting for tests to complete, they can adjust test parameters based on early results, maximizing learning opportunities and minimizing resource waste.
The technology also helps identify the most promising test variations before full deployment. This reduces the risk of negative impacts on your marketing performance while accelerating the pace of optimization.
Implementing Advanced Analytics Successfully
Success with advanced analytics requires careful planning and execution. Start by ensuring your data infrastructure can support these sophisticated techniques. This means having clean, well-organized data and appropriate processing capabilities.
Team training becomes crucial at this stage. While AI handles the heavy lifting of data analysis, your team needs to understand how to interpret and act on the insights generated. Regular training sessions and clear documentation help build this capability.
Create a framework for evaluating and implementing new analytics techniques. Not every advanced capability will provide value for your specific situation. Assess potential applications based on their alignment with your business objectives and resource requirements.
Future-Proofing Your Advanced Analytics Strategy
The field of AI analytics continues to evolve rapidly. Stay informed about emerging techniques and their potential applications in your marketing strategy. Regular evaluation of new capabilities helps ensure you maintain a competitive edge.
Build flexibility into your analytics infrastructure to accommodate new techniques and data sources. This might mean choosing platforms with robust API capabilities or maintaining clean, well-documented data structures that can support new analysis methods.
Remember that advanced analytics should serve your business objectives rather than becoming an end in themselves. Focus on applications that deliver measurable value and align with your overall marketing strategy.
Measuring Success and ROI
Implement clear metrics for evaluating the success of your advanced analytics initiatives. Track both direct performance improvements (like increased conversion rates or better campaign ROI) and indirect benefits (like faster decision-making or improved customer insights).
Create feedback loops that help refine and improve your analytics processes over time. Regular reviews of your analytics performance help identify areas for improvement and ensure continued alignment with business objectives.
By thoughtfully implementing these advanced techniques while maintaining focus on business value, you can build a sophisticated analytics capability that drives meaningful marketing improvements and sustainable competitive advantage.
Implementing AI Marketing Analytics Strategy
Creating an effective AI marketing analytics strategy requires careful planning, clear objectives, and a structured approach to implementation. This comprehensive guide will help you navigate the complex process of integrating AI analytics into your marketing operations.
Creating Your AI-Driven Marketing Roadmap
The journey toward AI-powered marketing begins with a clear roadmap. Start by assessing your current marketing analytics capabilities and identifying gaps that AI could fill. Consider your organization’s maturity level with data analytics and set realistic goals for implementation.
Your roadmap should outline specific phases of implementation, beginning with foundational elements like data collection and integration. Each phase should have clear objectives, timelines, and success metrics. This structured approach helps manage expectations and ensures steady progress toward your goals.
Remember to account for both technical and organizational changes in your roadmap. AI implementation often requires adjustments to existing processes and workflows. Plan for these changes to minimize disruption and maximize adoption.
Data Privacy and Compliance Considerations
Data protection must be a cornerstone of your AI marketing strategy in today’s privacy-conscious environment. Start by understanding the regulatory requirements that affect your business, including GDPR, CCPA, and industry-specific regulations.
Implement privacy by design principles in your AI analytics infrastructure. This means building privacy considerations into your data collection and analysis processes from the ground up rather than adding them as an afterthought. Consider data minimization, purpose limitation, and storage restrictions.
Create clear policies for data handling and ensure all team members understand their responsibilities. Document your data processing activities and maintain records of compliance measures. Regular audits help ensure ongoing compliance and identify potential issues before they become problems.
Building Team Capabilities
Success with AI marketing analytics requires more than just technology – it requires skilled people who can leverage these AI Marketing tools effectively. Begin by assessing your team’s current capabilities and identifying skill gaps that need to be addressed.
Develop a comprehensive training program that covers both technical and strategic aspects of AI analytics. Include topics like data interpretation, strategic application of insights, and ethical considerations in AI usage. Remember that training should be ongoing as technologies and capabilities evolve.
Consider creating specialized roles or teams focused on AI analytics. These might include data scientists, analytics specialists, or AI strategy coordinators. Clear role definitions and responsibilities help ensure effective implementation and ongoing management of your AI initiatives.
Integration with Existing Marketing Tools
Successful AI implementation requires seamless integration with your existing marketing technology stack. Begin by mapping out your current tools and understanding how AI analytics will interact with each component. This includes CRM systems, marketing automation platforms, content management systems, and other marketing tools.
Develop a clear integration strategy that minimizes disruption to ongoing operations. Consider using API connections where possible to ensure smooth data flow between systems. Plan for potential technical challenges and have contingency plans ready.
Test integrations thoroughly before full deployment. Start with small-scale pilots to identify and resolve issues early. Gradually expand the scope of integration as you confirm successful operation and user adoption.
Change Management and Adoption
The human element often determines the success or failure of AI implementations. Develop a comprehensive change management strategy that addresses the transition’s technical and cultural aspects.
Start by building buy-in at all levels of the organization. Communicate the benefits of AI analytics clearly and address concerns proactively. Share success stories and early wins to maintain momentum and enthusiasm for the initiative.
Create clear processes for handling resistance and addressing concerns. Establish feedback channels that allow team members to share their experiences and suggestions. Use this feedback to refine your implementation approach and improve adoption rates.
Resource Allocation and Management
Effective resource allocation plays a crucial role in successful AI implementation. Develop detailed budgets that account for all aspects of the initiative, including technology costs, training expenses, and ongoing maintenance requirements.
Consider both short-term and long-term resource needs. While initial implementation might require significant investment, plan for ongoing costs like software subscriptions, system updates, and continued training. Build flexibility to accommodate changing needs and emerging opportunities.
Establish clear metrics for measuring return on investment. Track both direct financial benefits and indirect improvements in efficiency and effectiveness. Use these metrics to justify continued investment and guide future resource allocation decisions.
Risk Management and Mitigation
Identify potential risks early in the implementation process and develop strategies to address them. Consider technical risks like data quality issues or integration problems, as well as organizational risks like resistance to change or skill gaps.
Create contingency plans for various scenarios that might impact your AI analytics implementation. This includes technical failures, data breaches, or changes in regulatory requirements. Regular risk assessments help identify emerging issues before they become serious problems.
Document your risk management procedures and ensure all team members understand their roles in risk mitigation. Regular reviews and updates to risk management strategies help maintain their effectiveness as your AI implementation matures.
Measuring Implementation Success
Establish clear metrics for measuring the success of your AI analytics implementation. Include technical metrics like system performance and business metrics like improved marketing outcomes. Regular monitoring of these metrics helps identify areas for improvement and demonstrates the value of your AI investment.
Create dashboards that provide visibility into implementation progress and results. Share these insights with stakeholders to maintain support for the initiative and guide future development. Use the data to refine your implementation strategy and improve outcomes over time.
Remember that success with AI marketing analytics is an ongoing journey rather than a destination. Continue to evaluate and adjust your approach as technologies evolve and your organization’s needs change. This flexible, iterative approach helps ensure long-term success with your AI analytics implementation.
AI Analytics for Customer Behavior
Understanding customer behavior has never been more critical or complex. AI analytics provides unprecedented insights into how customers think, act, and make decisions across their journey. Let’s explore how to leverage these powerful tools effectively.
Mapping the Modern Customer Journey
Today’s customer journey rarely follows a linear path. AI analytics helps decode these complex journeys by analyzing thousands of touchpoints simultaneously. The technology identifies patterns in customer interactions across multiple channels, from social media engagement to website behavior and purchase history.
Modern journey mapping goes beyond simple flowcharts. AI systems create dynamic maps that evolve in real-time based on customer actions. These maps reveal how different segments move through your marketing funnel, identifying common paths to purchase and potential dropout points.
Understanding these journey patterns helps optimize your marketing efforts. You might discover that customers who engage with specific content combinations are more likely to convert or that certain touchpoint sequences lead to higher customer lifetime value. These insights enable more effective resource allocation and campaign planning.
Behavioral Pattern Recognition
AI excels at identifying subtle patterns in customer behavior that humans might miss. The technology analyzes vast amounts of data to recognize behavioral signatures that indicate purchase intent, churn risk, or other significant outcomes.
These patterns often combine multiple factors. For instance, changes in browsing behavior, email engagement, and support interactions might signal an impending purchase decision together. AI systems can identify these complex patterns and trigger appropriate marketing responses automatically.
Pattern recognition also helps identify emerging trends before they become obvious. By analyzing changes in behavioral patterns across your customer base, AI can spot shifting preferences or new opportunities early enough to give you a competitive advantage.
Sentiment Analysis and Emotional Tracking
Understanding customer emotions is crucial for effective marketing. AI-powered sentiment analysis goes beyond simple positive/negative classifications to understand subtle emotional nuances in customer communications.
Modern sentiment analysis systems consider context and tone, not just keywords. They can detect sarcasm, identify emotional intensity, and understand industry-specific terminology. This deeper understanding helps create more effective, empathetic marketing communications.
Real-time emotional tracking allows for immediate response to customer feedback. If sentiment analysis detects growing frustration with a particular product feature or service aspect, you can address the issue before it impacts customer satisfaction and retention.
Purchase Prediction Models
AI analytics can predict purchase behavior with remarkable accuracy by analyzing patterns in customer data. These predictions help optimize inventory management, personalize marketing messages, and improve campaign timing.
Purchase prediction models consider numerous factors, including browsing history, past purchases, seasonal trends, and external events. They can identify when customers are most likely to buy and what products they’re most likely to be interested in.
These models become more accurate over time as they learn from new data. They can adjust their predictions based on changing customer behaviors and market conditions, helping you stay ahead of evolving customer needs.
Churn Prevention Analytics
Identifying and preventing customer churn becomes more sophisticated with AI analytics. The technology can spot early warning signs of customer dissatisfaction and predict potential churners before they leave.
Churn prevention models analyze multiple indicators, including changes in engagement patterns, support interactions, and product usage. They assign risk scores to customers and recommend specific interventions based on individual circumstances.
Automated alert systems can notify your team when high-value customers show churn risk indicators. This early warning system allows for proactive intervention, often preventing churn before it occurs.
Personalizing Customer Experiences
AI analytics enables personalization at scale by understanding individual customer preferences and behaviors. The technology can predict which content, products, or offers will resonate with specific customers at particular times.
Dynamic personalization adjusts in real-time based on customer behavior. If a customer’s browsing pattern suggests a shift in interests, the system can immediately update recommendations and content offerings to match.
Privacy and Ethical Considerations
While gathering detailed behavioral data, maintain strong privacy practices. Be transparent about data collection and usage. Implement strong data protection measures and ensure compliance with relevant regulations.
Consider ethical implications when using behavioral insights. Avoid manipulative practices and respect customer privacy preferences. Build trust by using behavioral data to create genuine value for customers rather than just maximizing short-term gains.
Regular privacy audits help ensure your behavioral analytics practices remain ethical and compliant. Document your data handling procedures and maintain clear policies for using behavioral insights.
Measuring Success
Define clear metrics for measuring the impact of your behavioral analytics initiatives. Track both direct outcomes like conversion rates and indirect benefits like improved customer satisfaction scores.
Create feedback loops that help refine your behavioral analytics models over time. Regular performance reviews help identify areas for improvement and ensure your analytics continue to deliver value.
Future Trends in AI Marketing Analytics
The landscape of AI marketing analytics continues to evolve at a rapid pace. Understanding emerging trends and preparing for future developments helps organizations maintain competitive advantage and maximize the value of their analytics investments.
Emerging Technologies Reshaping Marketing Analytics
Deep learning networks are revolutionizing how we understand customer behavior. These sophisticated systems can process unstructured data like images, voice, and video, opening new channels for customer insight. Marketing teams can now analyze customer interactions across multiple sensory dimensions, creating a more nuanced understanding of customer preferences and behaviors.
Edge computing brings AI analytics closer to data sources, enabling real-time processing and faster response times. This technology allows for immediate analysis of customer interactions, even in bandwidth-limited environments. Retail environments can process customer behavior data instantly, delivering personalized experiences without latency.
Quantum computing, while still emerging, promises to transform complex marketing analytics tasks. This technology could revolutionize optimization problems, enabling marketers to process vast amounts of data and consider countless variables simultaneously. The ability to analyze multiple scenarios in parallel could make current predictive models seem primitive by comparison.
Automated Decision Making Systems
Marketing automation is evolving toward autonomous decision-making systems. These platforms will provide insights and actively make and implement marketing decisions within defined parameters. This evolution requires a careful balance between automation and human oversight.
Real-time optimization systems will become more sophisticated, adjusting marketing efforts instantly based on changing conditions. These systems will consider multiple factors simultaneously, from weather patterns to stock market fluctuations, to optimize marketing messages and timing.
Predictive analytics will evolve into prescriptive systems that forecast outcomes and recommend specific actions to achieve desired results. These systems will consider complex interactions between different marketing channels and tactics to suggest optimal strategies.
Integration of Emerging Data Sources
Internet of Things (IoT) devices will provide new streams of customer behavior data. Smart homes, wearables, and connected vehicles offer customer habits and preferences insights. Marketing analytics platforms must evolve to effectively process and analyze these diverse data streams.
Augmented and virtual reality platforms will generate new types of interaction data. Understanding how customers behave in virtual environments will become increasingly important for marketers. Analytics systems must adapt to process and interpret these new forms of engagement data.
Voice interfaces will continue to grow in importance, generating valuable conversational data. Natural language processing capabilities will evolve to extract deeper insights from voice interactions, understanding not just what customers say but how they say it.
Conclusion
You’ve now got a solid foundation in AI marketing analytics, from basic concepts to advanced implementation strategies. I’ve shown you how to harness predictive analytics, optimize customer behavior tracking, and implement AI-driven decision-making in your marketing operations. Remember – success with AI isn’t about having the most sophisticated AI marketing tools; it’s about using them strategically to solve your specific marketing challenges.
Start small, but think big. Pick one area of your marketing that needs improvement and apply what you’ve learned. Maybe it’s customer segmentation, campaign optimization, or predictive analytics. Test, measure, and refine your approach. As you get comfortable with one aspect, gradually expand your AI implementation to other areas.
I encourage you to keep learning and experimenting with AI marketing analytics. The field is constantly evolving, and staying current with new developments will give you a competitive edge. Focus on creating value for your customers while building your AI capabilities. You’ve got the knowledge – now it’s time to put it into action and transform your marketing strategy.
Frequently Asked Questions (FAQs)
The investment varies based on your business size and needs. For small businesses, entry-level AI marketing tools typically range from $500-$2000 monthly, covering basic features like customer segmentation and campaign optimization. Enterprise solutions can range from $5000-$25000 monthly, offering comprehensive analytics capabilities. Beyond software costs, consider allocating a budget for team training, data infrastructure upgrades, and potential consulting support during implementation. Most vendors offer tiered pricing models that allow you to start small and scale up as your needs grow.
You’ll typically start seeing initial insights within 2-4 weeks of proper implementation. However, meaningful results that impact business outcomes usually take 3-6 months to materialize. This timeline allows the AI system to gather sufficient data, learn from patterns, and refine its predictions. More complex applications like customer lifetime value prediction may take 6-12 months to provide reliable insights. The key is to set realistic expectations and focus on incremental improvements rather than immediate transformational results.
While deep technical expertise isn’t required, your team should understand basic data analysis and interpretation, marketing metrics and KPIs, and campaign management principles. Basic statistical concepts and project management fundamentals are also helpful. Most vendors provide comprehensive training programs to build necessary skills. The key is having team members who are analytical, willing to learn, and able to translate data insights into marketing actions. Consider starting with a small, dedicated team and gradually expanding capabilities across your marketing organization
Modern AI platforms offer flexible integration options through API connections to major marketing platforms and pre-built integrations with common CRM systems. Custom integration capabilities handle proprietary systems, while data import/export functionality ensures smooth data flow. Real-time data synchronization keeps all systems updated. Integration typically takes 2-8 weeks, depending on your technical infrastructure. Most vendors provide integration support and documentation to ensure smooth implementation.
Maintaining AI marketing analytics involves regular system performance reviews, model retraining, and optimization. Monthly performance checks help identify potential issues early. Quarterly model retraining ensures accuracy as market conditions change. Data quality checks should occur regularly, along with privacy and compliance updates. Ongoing team training helps maximize system value. Expect to spend 5-10 hours monthly on basic maintenance tasks, with additional time needed for major updates or new feature implementations.